5. Dataset loading utilities¶
The sklearn.datasets package embeds some small toy datasets as introduced in the Getting Started section.
To evaluate the impact of the scale of the dataset (n_samples and n_features) while controlling the statistical properties of the data (typically the correlation and informativeness of the features), it is also possible to generate synthetic data.
This package also features helpers to fetch larger datasets commonly used by the machine learning community to benchmark algorithm on data that comes from the ‘real world’.
5.1. General dataset API¶
There are three distinct kinds of dataset interfaces for different types of datasets. The simplest one is the interface for sample images, which is described below in the Sample images section.
The dataset generation functions and the svmlight loader share a simplistic interface, returning a tuple (X, y) consisting of a n_samples x n_features numpy array X and an array of length n_samples containing the targets y.
The toy datasets as well as the ‘real world’ datasets and the datasets fetched from mldata.org have more sophisticated structure. These functions return a dictionary-like object holding at least two items: an array of shape n_samples * `` n_features`` with key data (except for 20newsgroups) and a NumPy array of length n_samples, containing the target values, with key target.
The datasets also contain a description in DESCR and some contain feature_names and target_names. See the dataset descriptions below for details.
5.2. Toy datasets¶
scikit-learn comes with a few small standard datasets that do not require to download any file from some external website.
load_boston() | Load and return the boston house-prices dataset (regression). |
load_iris() | Load and return the iris dataset (classification). |
load_diabetes() | Load and return the diabetes dataset (regression). |
load_digits([n_class]) | Load and return the digits dataset (classification). |
load_linnerud() | Load and return the linnerud dataset (multivariate regression). |
These datasets are useful to quickly illustrate the behavior of the various algorithms implemented in the scikit. They are however often too small to be representative of real world machine learning tasks.
5.3. Sample images¶
The scikit also embed a couple of sample JPEG images published under Creative Commons license by their authors. Those image can be useful to test algorithms and pipeline on 2D data.
load_sample_images() | Load sample images for image manipulation. |
load_sample_image(image_name) | Load the numpy array of a single sample image |
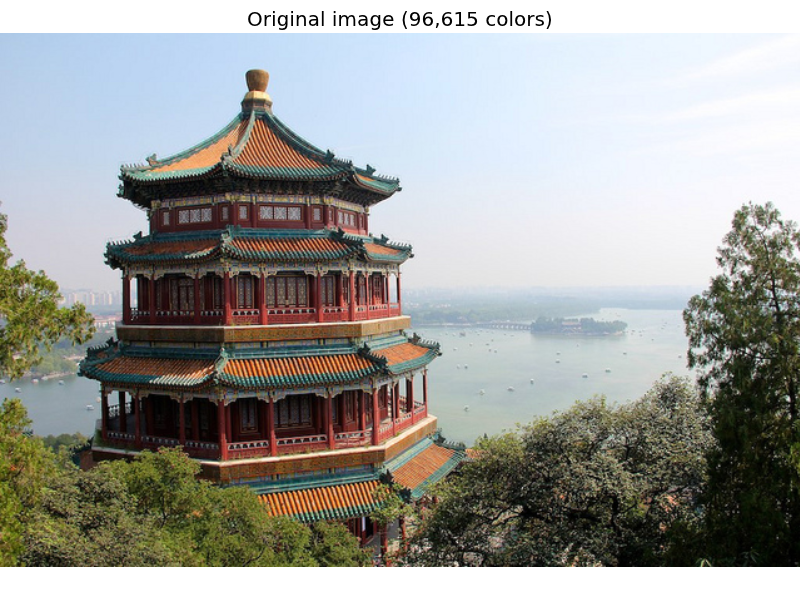
Warning
The default coding of images is based on the uint8 dtype to spare memory. Often machine learning algorithms work best if the input is converted to a floating point representation first. Also, if you plan to use pylab.imshow don’t forget to scale to the range 0 - 1 as done in the following example.
Examples:
5.4. Sample generators¶
In addition, scikit-learn includes various random sample generators that can be used to build artificial datasets of controlled size and complexity.
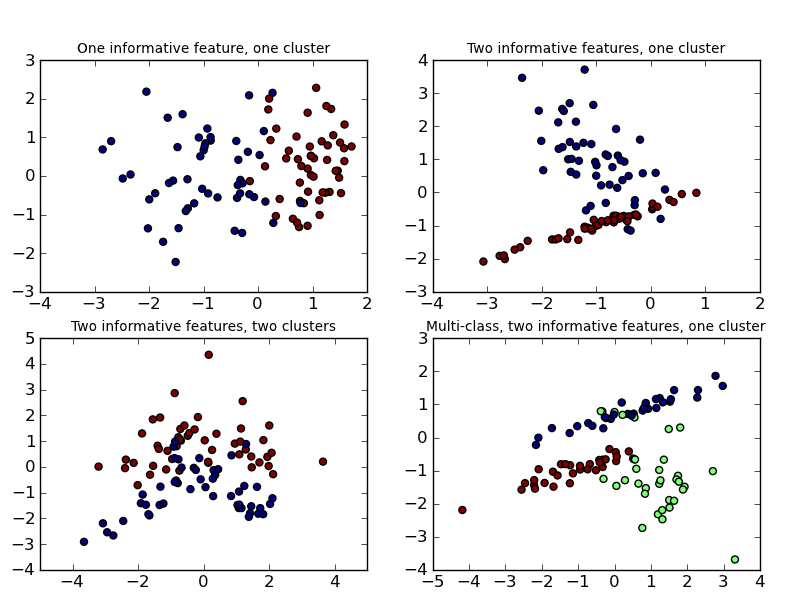
make_classification([n_samples, n_features, ...]) | Generate a random n-class classification problem. |
make_multilabel_classification([n_samples, ...]) | Generate a random multilabel classification problem. |
make_regression([n_samples, n_features, ...]) | Generate a random regression problem. |
make_blobs([n_samples, n_features, centers, ...]) | Generate isotropic Gaussian blobs for clustering. |
make_friedman1([n_samples, n_features, ...]) | Generate the “Friedman #1” regression problem |
make_friedman2([n_samples, noise, random_state]) | Generate the “Friedman #2” regression problem |
make_friedman3([n_samples, noise, random_state]) | Generate the “Friedman #3” regression problem |
make_hastie_10_2([n_samples, random_state]) | Generates data for binary classification used in |
make_low_rank_matrix([n_samples, ...]) | Generate a mostly low rank matrix with bell-shaped singular values |
make_sparse_coded_signal(n_samples, ...[, ...]) | Generate a signal as a sparse combination of dictionary elements. |
make_sparse_uncorrelated([n_samples, ...]) | Generate a random regression problem with sparse uncorrelated design |
make_spd_matrix(n_dim[, random_state]) | Generate a random symmetric, positive-definite matrix. |
make_swiss_roll([n_samples, noise, random_state]) | Generate a swiss roll dataset. |
make_s_curve([n_samples, noise, random_state]) | Generate an S curve dataset. |
make_sparse_spd_matrix([dim, alpha, ...]) | Generate a sparse symmetric definite positive matrix. |
make_biclusters(shape, n_clusters[, noise, ...]) | Generate an array with constant block diagonal structure for biclustering. |
make_checkerboard(shape, n_clusters[, ...]) | Generate an array with block checkerboard structure for biclustering. |
5.5. Datasets in svmlight / libsvm format¶
scikit-learn includes utility functions for loading datasets in the svmlight / libsvm format. In this format, each line takes the form <label> <feature-id>:<feature-value> <feature-id>:<feature-value> .... This format is especially suitable for sparse datasets. In this module, scipy sparse CSR matrices are used for X and numpy arrays are used for y.
You may load a dataset like as follows:
>>> from sklearn.datasets import load_svmlight_file
>>> X_train, y_train = load_svmlight_file("/path/to/train_dataset.txt")
...
You may also load two (or more) datasets at once:
>>> X_train, y_train, X_test, y_test = load_svmlight_files(
... ("/path/to/train_dataset.txt", "/path/to/test_dataset.txt"))
...
In this case, X_train and X_test are guaranteed to have the same number of features. Another way to achieve the same result is to fix the number of features:
>>> X_test, y_test = load_svmlight_file(
... "/path/to/test_dataset.txt", n_features=X_train.shape[1])
...
Related links:
Public datasets in svmlight / libsvm format: http://www.csie.ntu.edu.tw/~cjlin/libsvmtools/datasets/
Faster API-compatible implementation: https://github.com/mblondel/svmlight-loader
5.6. The Olivetti faces dataset¶
This dataset contains a set of face images taken between April 1992 and April 1994 at AT&T Laboratories Cambridge. The website describing the original dataset is now defunct, but archived copies can be accessed through the Internet Archive’s Wayback Machine.
As described on the original website:
There are ten different images of each of 40 distinct subjects. For some subjects, the images were taken at different times, varying the lighting, facial expressions (open / closed eyes, smiling / not smiling) and facial details (glasses / no glasses). All the images were taken against a dark homogeneous background with the subjects in an upright, frontal position (with tolerance for some side movement).
The image is quantized to 256 grey levels and stored as unsigned 8-bit integers; the loader will convert these to floating point values on the interval [0, 1], which are easier to work with for many algorithms.
The “target” for this database is an integer from 0 to 39 indicating the identity of the person pictured; however, with only 10 examples per class, this relatively small dataset is more interesting from an unsupervised or semi-supervised perspective.
The original dataset consisted of 92 x 112, while the version available here consists of 64x64 images.
When using these images, please give credit to AT&T Laboratories Cambridge.
5.7. The 20 newsgroups text dataset¶
The 20 newsgroups dataset comprises around 18000 newsgroups posts on 20 topics split in two subsets: one for training (or development) and the other one for testing (or for performance evaluation). The split between the train and test set is based upon a messages posted before and after a specific date.
This module contains two loaders. The first one, sklearn.datasets.fetch_20newsgroups, returns a list of the raw texts that can be fed to text feature extractors such as sklearn.feature_extraction.text.Vectorizer with custom parameters so as to extract feature vectors. The second one, sklearn.datasets.fetch_20newsgroups_vectorized, returns ready-to-use features, i.e., it is not necessary to use a feature extractor.
5.7.1. Usage¶
The sklearn.datasets.fetch_20newsgroups function is a data fetching / caching functions that downloads the data archive from the original 20 newsgroups website, extracts the archive contents in the ~/scikit_learn_data/20news_home folder and calls the sklearn.datasets.load_file on either the training or testing set folder, or both of them:
>>> from sklearn.datasets import fetch_20newsgroups
>>> newsgroups_train = fetch_20newsgroups(subset='train')
>>> from pprint import pprint
>>> pprint(list(newsgroups_train.target_names))
['alt.atheism',
'comp.graphics',
'comp.os.ms-windows.misc',
'comp.sys.ibm.pc.hardware',
'comp.sys.mac.hardware',
'comp.windows.x',
'misc.forsale',
'rec.autos',
'rec.motorcycles',
'rec.sport.baseball',
'rec.sport.hockey',
'sci.crypt',
'sci.electronics',
'sci.med',
'sci.space',
'soc.religion.christian',
'talk.politics.guns',
'talk.politics.mideast',
'talk.politics.misc',
'talk.religion.misc']
The real data lies in the filenames and target attributes. The target attribute is the integer index of the category:
>>> newsgroups_train.filenames.shape
(11314,)
>>> newsgroups_train.target.shape
(11314,)
>>> newsgroups_train.target[:10]
array([12, 6, 9, 8, 6, 7, 9, 2, 13, 19])
It is possible to load only a sub-selection of the categories by passing the list of the categories to load to the fetch_20newsgroups function:
>>> cats = ['alt.atheism', 'sci.space']
>>> newsgroups_train = fetch_20newsgroups(subset='train', categories=cats)
>>> list(newsgroups_train.target_names)
['alt.atheism', 'sci.space']
>>> newsgroups_train.filenames.shape
(1073,)
>>> newsgroups_train.target.shape
(1073,)
>>> newsgroups_train.target[:10]
array([1, 1, 1, 0, 1, 0, 0, 1, 1, 1])
5.7.2. Converting text to vectors¶
In order to feed predictive or clustering models with the text data, one first need to turn the text into vectors of numerical values suitable for statistical analysis. This can be achieved with the utilities of the sklearn.feature_extraction.text as demonstrated in the following example that extract TF-IDF vectors of unigram tokens from a subset of 20news:
>>> from sklearn.feature_extraction.text import TfidfVectorizer
>>> categories = ['alt.atheism', 'talk.religion.misc',
... 'comp.graphics', 'sci.space']
>>> newsgroups_train = fetch_20newsgroups(subset='train',
... categories=categories)
>>> vectorizer = TfidfVectorizer()
>>> vectors = vectorizer.fit_transform(newsgroups_train.data)
>>> vectors.shape
(2034, 34118)
The extracted TF-IDF vectors are very sparse, with an average of 159 non-zero components by sample in a more than 30000-dimensional space (less than .5% non-zero features):
>>> vectors.nnz / float(vectors.shape[0])
159.01327433628319
sklearn.datasets.fetch_20newsgroups_vectorized is a function which returns ready-to-use tfidf features instead of file names.
5.7.3. Filtering text for more realistic training¶
It is easy for a classifier to overfit on particular things that appear in the 20 Newsgroups data, such as newsgroup headers. Many classifiers achieve very high F-scores, but their results would not generalize to other documents that aren’t from this window of time.
For example, let’s look at the results of a multinomial Naive Bayes classifier, which is fast to train and achieves a decent F-score:
>>> from sklearn.naive_bayes import MultinomialNB
>>> from sklearn import metrics
>>> newsgroups_test = fetch_20newsgroups(subset='test',
... categories=categories)
>>> vectors_test = vectorizer.transform(newsgroups_test.data)
>>> clf = MultinomialNB(alpha=.01)
>>> clf.fit(vectors, newsgroups_train.target)
>>> pred = clf.predict(vectors_test)
>>> metrics.f1_score(newsgroups_test.target, pred)
0.88251152461278892
(The example Classification of text documents using sparse features shuffles the training and test data, instead of segmenting by time, and in that case multinomial Naive Bayes gets a much higher F-score of 0.88. Are you suspicious yet of what’s going on inside this classifier?)
Let’s take a look at what the most informative features are:
>>> import numpy as np
>>> def show_top10(classifier, vectorizer, categories):
... feature_names = np.asarray(vectorizer.get_feature_names())
... for i, category in enumerate(categories):
... top10 = np.argsort(classifier.coef_[i])[-10:]
... print("%s: %s" % (category, " ".join(feature_names[top10])))
...
>>> show_top10(clf, vectorizer, newsgroups_train.target_names)
alt.atheism: sgi livesey atheists writes people caltech com god keith edu
comp.graphics: organization thanks files subject com image lines university edu graphics
sci.space: toronto moon gov com alaska access henry nasa edu space
talk.religion.misc: article writes kent people christian jesus sandvik edu com god
You can now see many things that these features have overfit to:
- Almost every group is distinguished by whether headers such as NNTP-Posting-Host: and Distribution: appear more or less often.
- Another significant feature involves whether the sender is affiliated with a university, as indicated either by their headers or their signature.
- The word “article” is a significant feature, based on how often people quote previous posts like this: “In article [article ID], [name] <[e-mail address]> wrote:”
- Other features match the names and e-mail addresses of particular people who were posting at the time.
With such an abundance of clues that distinguish newsgroups, the classifiers barely have to identify topics from text at all, and they all perform at the same high level.
For this reason, the functions that load 20 Newsgroups data provide a parameter called remove, telling it what kinds of information to strip out of each file. remove should be a tuple containing any subset of ('headers', 'footers', 'quotes'), telling it to remove headers, signature blocks, and quotation blocks respectively.
>>> newsgroups_test = fetch_20newsgroups(subset='test',
... remove=('headers', 'footers', 'quotes'),
... categories=categories)
>>> vectors_test = vectorizer.transform(newsgroups_test.data)
>>> pred = clf.predict(vectors_test)
>>> metrics.f1_score(pred, newsgroups_test.target)
0.78409163025839435
This classifier lost over a lot of its F-score, just because we removed metadata that has little to do with topic classification. It loses even more if we also strip this metadata from the training data:
>>> newsgroups_train = fetch_20newsgroups(subset='train',
... remove=('headers', 'footers', 'quotes'),
... categories=categories)
>>> vectors = vectorizer.fit_transform(newsgroups_train.data)
>>> clf = BernoulliNB(alpha=.01)
>>> clf.fit(vectors, newsgroups_train.target)
>>> vectors_test = vectorizer.transform(newsgroups_test.data)
>>> pred = clf.predict(vectors_test)
>>> metrics.f1_score(newsgroups_test.target, pred)
0.73160869205141166
Some other classifiers cope better with this harder version of the task. Try running Sample pipeline for text feature extraction and evaluation with and without the --filter option to compare the results.
Recommendation
When evaluating text classifiers on the 20 Newsgroups data, you should strip newsgroup-related metadata. In scikit-learn, you can do this by setting remove=('headers', 'footers', 'quotes'). The F-score will be lower because it is more realistic.
5.8. Downloading datasets from the mldata.org repository¶
mldata.org is a public repository for machine learning data, supported by the PASCAL network .
The sklearn.datasets package is able to directly download data sets from the repository using the function fetch_mldata(dataname).
For example, to download the MNIST digit recognition database:
>>> from sklearn.datasets import fetch_mldata
>>> mnist = fetch_mldata('MNIST original', data_home=custom_data_home)
The MNIST database contains a total of 70000 examples of handwritten digits of size 28x28 pixels, labeled from 0 to 9:
>>> mnist.data.shape
(70000, 784)
>>> mnist.target.shape
(70000,)
>>> np.unique(mnist.target)
array([ 0., 1., 2., 3., 4., 5., 6., 7., 8., 9.])
After the first download, the dataset is cached locally in the path specified by the data_home keyword argument, which defaults to ~/scikit_learn_data/:
>>> os.listdir(os.path.join(custom_data_home, 'mldata'))
['mnist-original.mat']
Data sets in mldata.org do not adhere to a strict naming or formatting convention. fetch_mldata is able to make sense of the most common cases, but allows to tailor the defaults to individual datasets:
The data arrays in mldata.org are most often shaped as (n_features, n_samples). This is the opposite of the scikit-learn convention, so fetch_mldata transposes the matrix by default. The transpose_data keyword controls this behavior:
>>> iris = fetch_mldata('iris', data_home=custom_data_home) >>> iris.data.shape (150, 4) >>> iris = fetch_mldata('iris', transpose_data=False, ... data_home=custom_data_home) >>> iris.data.shape (4, 150)
For datasets with multiple columns, fetch_mldata tries to identify the target and data columns and rename them to target and data. This is done by looking for arrays named label and data in the dataset, and failing that by choosing the first array to be target and the second to be data. This behavior can be changed with the target_name and data_name keywords, setting them to a specific name or index number (the name and order of the columns in the datasets can be found at its mldata.org under the tab “Data”:
>>> iris2 = fetch_mldata('datasets-UCI iris', target_name=1, data_name=0, ... data_home=custom_data_home) >>> iris3 = fetch_mldata('datasets-UCI iris', target_name='class', ... data_name='double0', data_home=custom_data_home)
5.9. The Labeled Faces in the Wild face recognition dataset¶
This dataset is a collection of JPEG pictures of famous people collected over the internet, all details are available on the official website:
Each picture is centered on a single face. The typical task is called Face Verification: given a pair of two pictures, a binary classifier must predict whether the two images are from the same person.
An alternative task, Face Recognition or Face Identification is: given the picture of the face of an unknown person, identify the name of the person by referring to a gallery of previously seen pictures of identified persons.
Both Face Verification and Face Recognition are tasks that are typically performed on the output of a model trained to perform Face Detection. The most popular model for Face Detection is called Viola-Jones and is implemented in the OpenCV library. The LFW faces were extracted by this face detector from various online websites.
5.9.1. Usage¶
scikit-learn provides two loaders that will automatically download, cache, parse the metadata files, decode the jpeg and convert the interesting slices into memmaped numpy arrays. This dataset size is more than 200 MB. The first load typically takes more than a couple of minutes to fully decode the relevant part of the JPEG files into numpy arrays. If the dataset has been loaded once, the following times the loading times less than 200ms by using a memmaped version memoized on the disk in the ~/scikit_learn_data/lfw_home/ folder using joblib.
The first loader is used for the Face Identification task: a multi-class classification task (hence supervised learning):
>>> from sklearn.datasets import fetch_lfw_people
>>> lfw_people = fetch_lfw_people(min_faces_per_person=70, resize=0.4)
>>> for name in lfw_people.target_names:
... print(name)
...
Ariel Sharon
Colin Powell
Donald Rumsfeld
George W Bush
Gerhard Schroeder
Hugo Chavez
Tony Blair
The default slice is a rectangular shape around the face, removing most of the background:
>>> lfw_people.data.dtype
dtype('float32')
>>> lfw_people.data.shape
(1288, 1850)
>>> lfw_people.images.shape
(1288, 50, 37)
Each of the 1140 faces is assigned to a single person id in the target array:
>>> lfw_people.target.shape
(1288,)
>>> list(lfw_people.target[:10])
[5, 6, 3, 1, 0, 1, 3, 4, 3, 0]
The second loader is typically used for the face verification task: each sample is a pair of two picture belonging or not to the same person:
>>> from sklearn.datasets import fetch_lfw_pairs
>>> lfw_pairs_train = fetch_lfw_pairs(subset='train')
>>> list(lfw_pairs_train.target_names)
['Different persons', 'Same person']
>>> lfw_pairs_train.pairs.shape
(2200, 2, 62, 47)
>>> lfw_pairs_train.data.shape
(2200, 5828)
>>> lfw_pairs_train.target.shape
(2200,)
Both for the fetch_lfw_people and fetch_lfw_pairs function it is possible to get an additional dimension with the RGB color channels by passing color=True, in that case the shape will be (2200, 2, 62, 47, 3).
The fetch_lfw_pairs datasets is subdivided into 3 subsets: the development train set, the development test set and an evaluation 10_folds set meant to compute performance metrics using a 10-folds cross validation scheme.
References:
- Labeled Faces in the Wild: A Database for Studying Face Recognition in Unconstrained Environments. Gary B. Huang, Manu Ramesh, Tamara Berg, and Erik Learned-Miller. University of Massachusetts, Amherst, Technical Report 07-49, October, 2007.
5.9.2. Examples¶
5.10. Forest covertypes¶
The samples in this dataset correspond to 30×30m patches of forest in the US, collected for the task of predicting each patch’s cover type, i.e. the dominant species of tree. There are seven covertypes, making this a multiclass classification problem. Each sample has 54 features, described on the dataset’s homepage. Some of the features are boolean indicators, while others are discrete or continuous measurements.
sklearn.datasets.fetch_covtype will load the covertype dataset; it returns a dictionary-like object with the feature matrix in the data member and the target values in target. The dataset will be downloaded from the web if necessary.